Best Practices - Quality Assurance
Quality Assurance (QA)
The aim of Quality Assurance is to document and comment on evaluation methods together with suspicious results. The QA does not perform any corrections.
Quality Assurance of data plays an important role in the data publication process as well as for data re-use. However, quality control procedures and quality documentation vary greatly among scientific data. In general every project defines their quality procedures.
In order to make the different Quality Assessments of the projects comparable, a generic Quality Assessment System is under development. Based on the self-assessment approach of a maturity matrix, an objective and uniform quality level system for data is derived. It consists of 5 maturity quality levels, starting with the initial level=1.
What is the goal of the Quality Assessment System ?
- Encourage data creators to improve their quality assessment procedures to reach the next quality level.
- Enable data consumers to decide, whether a dataset has a quality that is sufficient for usage in the aimed application.
Quality Maturity Matrix
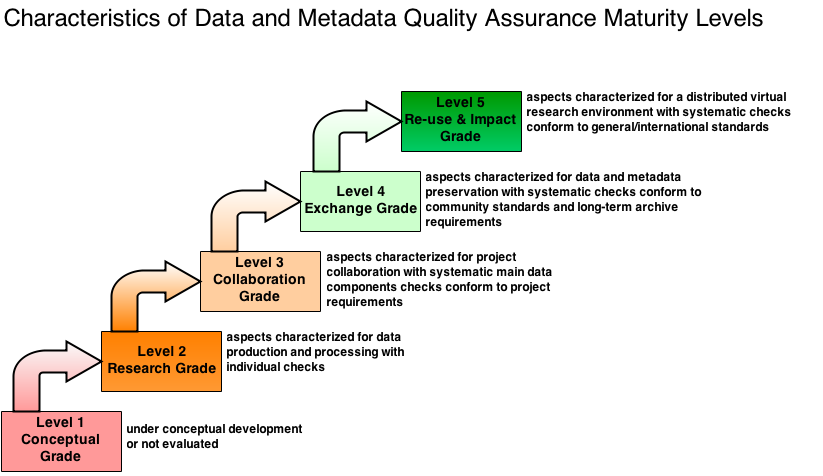
Different criteria are defined, which are subdivided into aspects. For every aspect the 5 maturity quality levels are defined.
The Criteria are (Version 06.11.2014):
Consistency
• Data Organisation and Data Object
• Versioning and Controlled Vocabularies (CVs)
• Data-Metadata Consistency
Completeness
• Existence of Data
• Existence of Core Metadata and Provenance
Accessibility
• Technical Data Access by Identifier/Lineage
• Core Metadata and Provenance Access by Identifier
Accuracy
• Plausibility
• Statistical Anomalies
State of the Quality Assessment System
The Quality Assessment System is currently developed. The information on this page will be updated as progress is made. Comments and suggestions are welcome.
Maturity Level Description (Version 06.11.2014):
Characteristics: under conceptual development or not evaluated
Score 1 is always reached
Characteristics: aspects characterized for data production and processing with individual checks
Score 2 rating scales correspond to the following aspects:
Consistency:
Data Organisation and Data Object:
- informal data organization is consistent
- file names are consistent to internal rules
- file extensions are consistent
Versioning and Controlled Vocabularies (CVs):
- informal versioning is consistent
- CVs are consistent
DataMetadata Consistency: creators are correct
Completeness:
Existence of Data: data is in production and may be deleted or overwritten
Existence of Core Metadata and Provenance:
- creators exist
- data provenance is unsystematically documented
Accessibility:
Technical Data Access by Identifier/Lineage: data is accessible by file names
Core Metadata and Provenance Access by Identifier:
- creators are accessible
- data provenance unsystematically documented is accessible
Accuracy:
Plausibility: documented procedure about technical sources of errors and deviation/inaccuracy exists
Statistical Anomalies: missing values are indicated e.g. with fill values
Characteristics: aspects characterized for project collaboration with systematic main data components checks conform to project requirements
Score 3 rating scales correspond to the following aspects:
Consistency:
Data Organisation and Data Object:
-
data organisation is documented
-
internal identifiers (with mapping to data objects) e.g. file names and formats correspond to project requirements
- file extensions, size and checksum of main components are consistent
Versioning and Controlled Vocabularies (CVs):
- systematic versioning correspond to project requirements
- formal CVs of main components are consistent
DataMetadata Consistency: creators/contact are correct
Completeness:
Existence of Data: datasets exist, not complete and may be deleted but not overwritten unless explicitly specified
Existence of Core Metadata and Provenance:
- creators/contact exist
- naming conventions for discovery exist
- datasets provenance is basically documented e.g. in data header
Accessibility:
Technical Data Access by Identifier/Lineage:
- datasets are accessible by internal identifier and mapping (bijectiv) to objects are documented e.g. in data header
- checksums are accessible
Core Metadata and Provenance Access by Identifier:
- creators/contact with naming conventions are accessible
- dataset provenance is accessible
Accuracy:
Plausibility:
- documented procedure about technical sources of errors and deviation/inaccuracy exists
- documented procedure about methodological sources of errors and deviation/inaccuracy exists
Statistical Anomalies:
- missing values are indicated e.g. with fill values
- documented procedure about rough anomalies are available e.g. outliers concerning limits
Characteristics: aspects characterized for data and metadata preservation with systematic checks conform to community standards and long-term archive requirements
Score 4 rating scales correspond to the following aspects:
Consistency:
Data Organisation and Data Object:
- data organization is structured/conform according to welldefined rules
- entry names and data formats are conform to community standards
- datasets are reusable with selfdescribing data objects which meet the community standards
- file extension, size and checksum are consistent
Versioning and Controlled Vocabularies (CVs):
- systematic versioning collection including documentation of enhancement is conform to community standards
- old versions stored if feasible
- formal CVs of data are conform to community standards
DataMetadata Consistency:
data source e.g. sensor are consistent creators/contact/publisher are consistent metadata for search and discovery e.g. keywords are consistent quality assurance procedure for data and metadata consistency (approval and review) is documented data citation metadata to a documented procedure are consistent
Completeness:
Existence of Data:
- data entities (conform to community standards) are complete (dynamic datasets - data stream are not affected)
- number of data sets (aggregation) is consistent
- data are persistent, as long as expiration date requires
Existence of Core Metadata (main components) and Provenance:
- data source e.g. sensor
- creators/contact/publisher
- metadata for search and discovery e.g. keywords
- quality assurance procedure (approval and review)
- data citation
- detailed description of data production steps and method
- data expiration date
- access constraint
Accessibility:
Technical Data Access by Identifier/Lineage:
- complete datasets (conform to community standards) are accessible by permanent (minimum 10 years see rules of good scientific practice) identifier with resolving to data access as long as expiration date requires
- checksums are accessible
Core Metadata and Provenance Access by Identifier:
- main metadata components:
-data source e.g. sensor
-creators/contact/publisher
-metadata for search and discovery e.g. keywords
-quality assurance procedure for data and metadata consistency (approval and review) is documented
-data citation metadata to a documented procedure are consistent
with data expiration date are accessible by identifier
- detailed description of data production steps and methods are accessible by identifier
Accuracy:
Plausibility:
- documented procedure about technical sources of errors and deviation/inaccuracy exists
- documented procedure about methodological sources of errors and deviation/inaccuracy exists
Statistical Anomalies:
- missing values are indicated e.g. with fill values
- documented procedure about rough anomalies are available e.g. outliers concerning limits.
- documented procedure about systematic deviations in time and space (e.g. changes in mean, variance and trends) and random errors exist
- scientific consistency among multiple data sets and their relationships is documented if feasible
Characteristics: aspects characterized for a distributed virtual research environment with checks conform to general/international standards
Score 5 rating scales correspond to the following aspects:
Consistency:
Data Organisation and Data Object:
- data organization is structured/conform according to standardized rules
- data formats are conform to general/international standards
- data objects are consistent to external scientific objects and up-to-date
- file extension, size and checksum are consistent
- data objects with general/international standards are self-describing
- data objects are fully machine-readable with references to sources
Versioning and Controlled Vocabularies (CVs):
- systematic versioning collection including documentation of enhancement is conform to community standards
- old versions stored if feasible
- documentation of not included newer versions is consistent
- CVs are general/international standardized
DataMetadata Consistency:
- data source e.g. sensor
- creators/contact/publisher
- metadata for search and discovery e.g. keywords
- quality assurance procedure for data and metadata consistency (approval and review) is documented
- data citation metadata to a documented procedure are consistent
- external metadata and data are consistent
Completeness:
Existence of Data:
- data entities (conform to general/international standards) are complete (dynamic datasets - data stream are not affected)
- number of data sets (aggregation) is consistent
- data are persistent, as long as expiration date requires
Existence of Core Metadata and Provenance:
- metadata is conform to general/international standards
- data provenance chain exists including internal and external objects e.g. software, articles, method and workflow description
Accessibility:
Technical Data Access by Identifier/Lineage:
- complete data (conform to general/international standards) is accessible by global resolvable identifier (PID) registered with resolving to data access including backup as long as expiration date requires
- data is accessible within other data infrastructures including cross references
- external PID references supported
- provenance chain is accessible
Core Metadata and Provenance Access by Identifier:
- metadata with data expiration date including backup general/international standardizedare accessible by global resolvable identifier
- data provenance chain including internal and external objects e.g. software, articles, methods and workflow description are accessible by global resolvable identifier
Accuracy:
Plausibility:
- documented procedure about technical sources of errors and deviation/inaccuracy exists
- documented procedure about methodological sources of errors and deviation/inaccuracy exists
- documented procedure with validation against independent data exists
- references to evaluation results (data) and methods exist
Statistical Anomalies:
- missing values are indicated e.g. with fill values
- documented procedure about rough anomalies are available e.g. outliers concerning limits
- documented procedure about systematic deviations in time and space (e.g. changes in mean, variance and trends) and random errors exist
- scientific consistency among multiple data sets and their relationships is documented if feasible
Checklists (in work):
Examples
Characteristics: under conceptual development or not evaluated
Score 1 is always reached.
To achieve a specific desired score level it would be helpful to have a data management plan. This should include the conceptual development.
Characteristics: aspects characterized for data production and processing with individual checks
Consistency:
- Is the directory structure correct?
- Are the file sizes feasible for access and transmission?
- Are the file names correct in accordance to CVs if applicable?
- Are the file extensions correct in accordance to format if feasible?
Checks: informal in accordance to Data Management Plan
- Is a versioning available if feasible?
- How is the versioning implemented if feasible?
- Are CVs applied if feasible?
Data-Metadata Consistency
Checks: informal
- Are creators correct?
Completeness:
Checks: informal
- Are creators documented?
- Is data provenance unsystematically documented?
Accessibility:
Checks: informal
- Is data accessible by file names?
Core Metadata and Provenance Access by Identifier:
Checks: informal
- How are creators and data provenance documentation available/accessible by identifier?
Accuracy:
Checks: informal
- Is an estimation of technical errors available?
- Detections of anomalies in model datasets with sources of errors and deviations e.g. technical (Appendix A 1.1)?
- Detections of anomalies in observational datasets with sources of errors and deviations:
- Is a classification into a level system available e.g. http://www.godae.org/Data-definition.html ?
Checks: spot checks required
- Are missing values indicated e.g. with fill values or in metadata?
The checks are documented in project and institute reports (paper, internet and articles). Checks are done and documented under control of project data management.
Characteristics: aspects characterized for project collaboration with systematic main data components checks conform to project requirements
Consistency:
- Is a documentation with minimal specification of data organization available?
- Is the directory structure correct?
- Are the file sizes feasible for access and transmission?
- Are the file names or internal identifiers with mapping to objects correct in accordance to CVs?
- Are the formats and file extensions correct (checked with format checker)?
- Are the data sizes checked and correct, the size of data set is not equal 0?
- Are checksums correct?
Checks: required for main data components
- Is a versioning available if feasible?
- How is the versioning implemented if feasible? If no explicit versioning exists an implicit versioning could be established. But a good way of versioning implementation is the identification with the date of storage. As soon as more than one version exists versioning should be implemented.
- Are CVs applied?
- Are formal CVs of standard components correct?
- Naming conventions for discovery e.g. CVs
Checks: required for main data components
- Are creators/contact e.g. in data headers correct?
Completeness:
Existence of Data
Checks: required for main data components
- How is data overwriting prevented? Removing is allowed and overwriting is allowed if explicitly specified
Checks: required for main data components
- Do metadata exist:
-creators/contact
-Keywords for discovery e.g. CVs - Is dataset provenance basically documented?
Accessibility:
Checks: required for main data components
- Are data accessible by internal identifier?
- Are internal identifiers with mapping to objects available?
- Are checksums accessible?
Checks: required for main data components
- Are creators/contact accessible by internal identifiers?
- Is dataset provenance accessible?
- How is the technical access implemented?
Accuracy:
Checks: required for main data components
Does an estimation of errors exist?
- Detections of anomalies in model dataset with sources of errors and deviations e.g. technical (Appendix A 1.1)
- Detections of anomalies in model dataset with methodological sources of errors and deviations.
- Detections of anomalies in observational dataset with sources of errors and deviations:Does a classification into a level system exist e.g. http://www.godae.org/Data-definition.html ?
- Detections of anomalies in observational dataset with methodological sources of errors and deviations.
Checks: required for main data components
- Are missing values indicated e.g. with fill values or in metadata?
- Are rough errors indicated (example tests see Appendix A 1.2)
The check procedure and check status are available at the repository. Checks are done and documented under control of repository.
Characteristics: aspects characterized for data and metadata preservation with systematic checks conform to community standards and long-term archive requirements
Consistency:
- Is a documentation with specification of well-defined rules of data organization available?
- Is the storage structure correct e.g. directory, access database tables?
- Are the file sizes feasible for access and transmission?
- Are the entry names correct in accordance to community standards?
- Are the data formats self-describing, conform to community standards and file extensions correct?
- Are the data size checked and correct, the size of data set is not equal 0?
- Are checksums correct?
Checks: required, systematic and conform to community standards and long-term archive requirements
- How is the systematic versioning on files or data collection implemented and applied?
If no explicit versioning exists an implicit versioning could be established. But a good way of versioning implementation is the identification with the date of storage. As soon as more than one version exists versioning should be implemented.
Are old data versions stored if feasible? - Are old data versions stored if feasible?
- Are formal CVs conform to community standards?
Checks: required, systematic and conform to community standards and long-term archive requirements
- Are metadata components to a documented procedure correct (components see Appendix A1.3)?
Completeness:
Existence of Data
Checks: required, systematic and conform to community standards and long-term archive requirements
- Are data entities complete (dynamic datasets – data stream are not effected)?
- Is the number of data sets correct in accordance to data management plan?
- Is data overwriting and removing of old versions prevented in accordance to expiration date?
Checks: required, systematic and conform to community standards and long-term archive requirements
- Are core metadata components (see Appendix A 1.3) to a documented procedure complete?
Accessibility:
Checks: required, systematic and conform to community standards and long-term archive requirements
- Is an expiration date of data available?
- Are permanent (as long as expiration date requires) identifier to data assigned?
- Are checksums accessible?
Checks: required, systematic and conform to community standards and long-term archive requirements
- Are core metadata components (see Appendix A 1.3) accessible by identifier?
- Are detailed description of data production steps and methods accessible by identifier?
Accuracy:
Checks: required, systematic and conform to community standards and long-term archive requirements
- Does an estimation of errors exist?
- Detections of anomalies in model dataset with sources of errors and deviations e.g. technical (Appendix A 1.1)
- Detections of anomalies in model dataset with methodological sources of errors and deviations.
- Detections of anomalies in observational dataset with sources of errors and deviations:
- Does a classification into a level system exist e.g. http://www.godae.org/Data-definition.html ?
- Detections of anomalies in observational dataset with methodological sources of errors and deviations.
Checks: required, systematic and conform to community standards and long-term archive requirements
- Are missing values indicated e.g. with fill values or in metadata?
- Are rough errors indicated (example tests see Appendix A 1.2)
- Is a documented procedure about shift in mean, variance and trends available?
- Is a documented procedure about random errors (inherent uncertainties of measurements see Appendix A 1.4) available?
Check procedure and check status are available and meet documentation standards at long-term archive with status of QA criteria. Checks are documented under control of long-term-archive.
Characteristics: aspects characterized for a distributed virtual research environment with checks conform to general/international standards
Consistency:
- Is a documentation with specification of standardized rules of data organization available?
- Is the storage structure correct e.g. directory, access database tables?
- Are the file sizes feasible for access and transmission?
- Are the entry names correct in accordance to standardization?
- Are the data formats self-describing, conform to general standards and file extensions correct?
- Are the data objects fully machine-readable with reference to sources?
- Are the data size checked and correct, the size of data set is not equal 0?
- Are checksums correct?
- Which external objects exist and are they consistent to data object?
Checks: required, systematic and conform to community standards and long-term archive requirements
- How is the systematic versioning on files or data collection implemented and applied?
If no explicit versioning exists an implicit versioning could be established. But a good way of versioning implementation is the identification with the date of storage. As soon as more than one version exists versioning should be implemented. - Are old data versions stored if feasible?
- Are formal CVs conform to international standards?
- Is the documentation of not included newer versions correct?
Checks: required, systematic and conform to community standards and long-term archive requirements
- Are metadata components to a documented procedure correct (components see Appendix A1.3)?
- Are external metadata and data consistent?
Completeness:
Existence of Data
Checks: required, systematic and conform to community standards and long-term archive requirements
- Are data entities complete (dynamic datasets – data stream are not effected)?
- Is the number of data sets correct in accordance to data management plan?
- Is data overwriting and removing of old versions prevented in accordance to expiration date?
Checks: required, systematic and conform to community standards and long-term archive requirements
- Are core metadata components (Appendix A 1.3) are complete and available with international standards like ISO 19135?
- Does a documentation of the data provenance chain exist?
Accessibility:
Checks: required, systematic and conform to community standards and long-term archive requirements
- Is an expiration date of data available?
- Are global resolvable, permanent (as long as expiration date requires) identifier (PIDs) to data assigned?
- Are checksums accessible?
- Is data accessible within other data infrastructures?
- Are cross-references supported?
- Is a data backup available?
- Is the documentation of the provenance chain accessible by identifier?
Checks: required, systematic and conform to community standards and long-term archive requirements
- Are general/standardized metadata accessible by global resolvable identifier?
- Are detailed description of data production steps and methods accessible by identifier?
- Is a backup of metadata accessible by global resolvable identifier or does a recovery system exist?
- Is a documentation of provenance chain including internal and external objects accessible by global resolvable identifier?
Accuracy:
Checks: required, systematic and conform to community standards and long-term archive requirements
- Does an estimation of errors exist?
-Detections of anomalies in model dataset with sources of errors and deviations e.g. technical (Appendix A 1.1)
-Detections of anomalies in model dataset with methodological sources of errors and deviations.
-Detections of anomalies in observational dataset with sources of errors and deviations:
-Does a classification into a level system exist e.g. http://www.godae.org/Data-definition.html ?
-Detections of anomalies in observational dataset with methodological sources of errors and deviations. - Is a documented procedure with validation against independent data available? E.g. Model Data in connection with model development see Appendix A 1.5
- Are references to the evaluation results with methods available?
Checks: required, systematic and conform to community standards and long-term archive requirements
- Are missing values indicated e.g. with fill values or in metadata?
- Are rough errors indicated (example tests see Appendix A 1.2)
- Is a documented procedure about shift in mean, variance and trends available?
- Is a documented procedure about random errors (inherent uncertainties of measurements see Appendix A 1.4) available?
Check procedure and check status are available and meet documentation standards at long-term archive with status of QA criteria. Checks are documented under control of long-term-archive.
1.1 Technical Sources of Errors and Deviations (not complete)
- source code
-Robustness in extrem values
-Exception for overflow and zero
-Used units e.g. mm/d or m/sec
-Errors like latitude, longitude, rotated pools grid conversion confusion
-Statistic problems – samples over time versus instantaneous values
-Units conversion and confusion
- Compiler
- Software library
- Number of processors
- Computer accuracy – numerical stability for very large and small values
1.2 Rough Errors Tests (Examples)
- LIM-test by Meek and Hatfield [94] are applied. (The test checks every data point on whether it exceeds a predefined range of values.)
- NOC-test by Meek and Hatfield [94] are applied. (The test checks on whether data does not change for more than a predefined number of values. It can be used to detect errors of instrument.)
- ROC-test by Meek and Hatfield [94] are applied. (The test checks the rate of change. The difference between two consecutive elements is checked concerning limits.)
1.3 Core Metadata Components
- source e.g. sensor (e.g. models: CLM 2.4.11 on NEC-SX6(hurrikan) or observational data: In Situ Land-based Platforms, GROUND STATIONS with In Situ/Laboratory Instruments)
- creators/contact/publisher
- Metadata for search and discovery if feasible:
-The time description (metadata) and data (in data header and file names) are consistent - calendric aspects, gaps, doubles, step widths
-The spatial description (metadata) and data (in data header and file names) are consistent (latitude, longitude, grid)
-The variable description with units (metadata) and data (in data header and file names) are consistent
-The description how the data have been determined or derived by the specified method e.g. statistics applying to data representation: samples over time - temporal means (e.g. monthly means), instantaneous – point is correct
-keywords
- Quality assurance procedure of approval and review
- Citation metadata (creators , publication date , title , publisher and persistent identifier)
- Description of data production steps and method description (project description and summary)
- Expiration date of data
- Access constraint
- Contributor(s) if feasible
1.4 Random Errors Observational Data
- Calibration of instruments
- Precise time and location description
- Situation in the field of measurement
- Physical description of the measured data
These descriptions are very detailed and should be published in a journal.
1.5 Model Data in connection with model development
- For the model data, these include the description of the models, components and their equations. This is also very detailed and should be published in a journal. E.g. http://www.geoscientific-model-development.net/ project or institute reports.
- Documentation with checklists of prognostic and diagnostic variables
- Description of simulation with:
-Parameterisation
-Resolution in time and space, dependencies of time and space resolutions (what happens if wind speed is stronger than the grid cell represents). Are there descriptions in table form?
-Boundary conditions
-Input data
-Constants – for initialization and run e.g. orography, solar constant, drag coefficient, area leaf index
- Description of family trees of models like: http://www.gfdl.noaa.gov/jrl_gcm or http://www.aip.org/history/climate/xAGCMtree.htm
References:
Meek, D. Hatfield, J. (1994) Data quality checking for single station meteorological databases.Agricultural and Forest Meteorology - AGR FOREST METEOROL , vol. 69, no. 1-2, pp. 85-109, DOI: 10.1016/0168-1923(94)90083-3
QA Documentation
ISO 19157 (in work)
DataCite (in work)