Data and Metadata
A good structure and documentation of your data ensures proper, efficient, and wide re-use of your data.
Metadata
Documentation on your data. Good data documentation includes detailed information on:
What: title, name
Data type description
Sensor – instrument, model
Source – platform, computer
Level e.g. http://www.godae.org/Data-definition.html
How: method, QA/QC and lineage: pre- and post processing, provenance
When
Where
Who
Why
Make data clear to understand and easy to use for:
Browse, Search and Retrieval
Ingest, Quality Assurance, Reprocessing
Application to Application Transfer
Storage and Archive
Re-use
Aggregated metadata is required by a number of services to the community as well as services to provide links to cited literature. These services, in turn, allow other initiatives (e.g. GEO, Future Earth, and similar) to draw on qualified metadata collections for their own applications and services.
One of the significant practical problems associated with meta-data aggregations relates to granularity of the data being described. The size and nature of data sets described in a meta-data record varies greatly between institutions, scale of observation, data families, and scientific disciplines. Furthermore, some data sets (for example sensor data) are updated continuously, and as such do not have a ‘beginning’ and an ‘end’.
Greatly differing granularity is not ideal in at least three cases: (1) search results derived from an aggregated meta-data collection are difficult to interpret, (2) automated processing of data sets is more difficult, and (3) not all data sets are easily citable.
In the following some askpects for choosing the appropriate granularity:
Technical
Unique Instrument, Survey, Experiment, or Platform
- A unique instrument/platform/project combination
Unique Parameter
- A unique parameter, parameter combination, or set of independent variables contained in the data set
Distinct Coverage
- Distinct temporal, spatial, or topic coverages
Versions/ Processing Level
- Data sets at varying stages of processing – specifically applicable to sensor data, but in a general sense distinguishing data at stages of raw observation, quality assured, and processed/ transformed data. Hence we are amending this to reflect the life cycle of the data set, and each significant life cycle stage or version should be uniquely citable.
Practical/ Functional
Size
- The sheer size of some data sets makes access to them impractical for most users. Hence one has to consider the case where a single data set is referenced by multiple meta-data records, ideally incorporating some for of sub-set resolving query to the data. The sub-setting criteria are similar to the ones discussed here.
Business Value
- Assigning unique identifiers to ever smaller data sub-sets loses business value at some point, hence there is a trade-off between the other considerations listed here and the cost of assigning a meta-data record and unique identifier.
Citability and Authorship
Body of Work
- The data supporting a logical body of work (journal article, research project) should have a separate meta-data record.
Unique Authorship
- A meta-data record should be split if the data being described has more than one unique set of authors.
Reproducibility
- This rule should allow scientists that seek to reproduce results to uniquely and easily find the data set(s) used for the original research.
full article: ICSU WDS (2014) Guidelines in Respect of Metadata Granularity, http://goo.gl/nhCDvK
Typically a data centre’s data deposit form or metdata editor are completed by the data creator
Ingest examples:
WDCC/CERA http://cera-www.dkrz.de/pls/apex/f?p=110:10:2317779178940753
CMIP5 Questionnaire http://q.cmip5.ceda.ac.uk/
DataCite API https://mds.datacite.org/static/apidoc
To view the list of KomFor data portals & service providers follow this link.
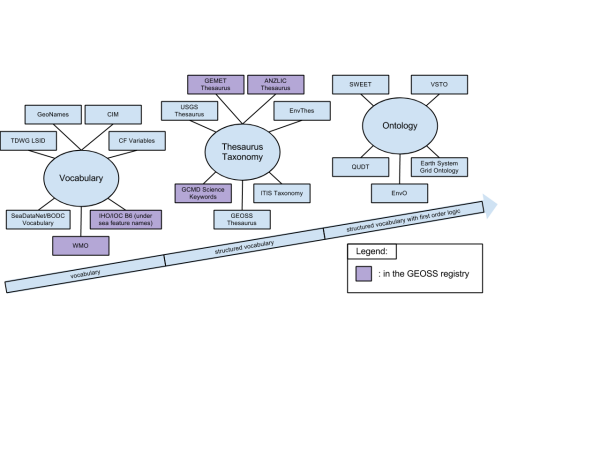
CMIP5 Data Reference Syntax (DRS) and Controlled Vocabularies
Entry name/title of data are specified according to the Data Reference Syntax (http://cmip-pcmdi.llnl.gov/cmip5/docs/cmip5_data_reference_syntax.pdf) as activity / product / institute / model / experiment / frequency / modeling realm / MIP table / ensemble member / version number / variable name / CMOR filename.nc.
Data
Data are digital information e.g. numbers, words, images, etc.
Standard formats ensures interchangeability and long-term usability.
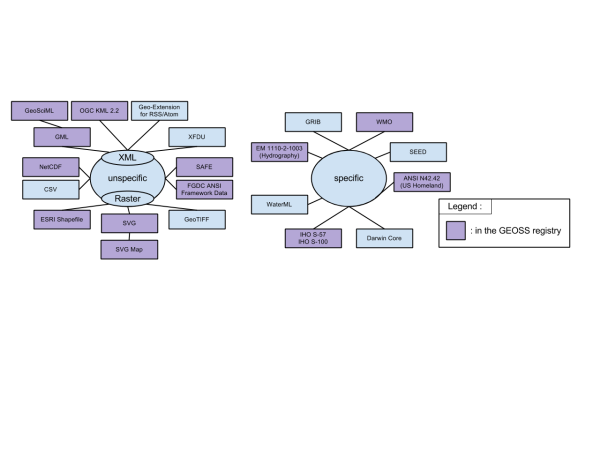
Examples of data standards:
cf-netcdf http://cf-pcmdi.llnl.gov/
WMO GRIB http://www.wmo.int/pages/prog/www/WMOCodes/Guides/GRIB/Introduction_GRIB1-GRIB2.pdf
Quality control and assurance takes place at various stages of research: data production / processing, ingest and long-term archiving, data publishing and dissemination
Dynamic datasets are transient. The rules of the change depends on different use cases.
Data Streams: data from monitoring services with continuous output
For datasets that are continuously and rapidly updated, there are special challenges both in citation and preservation. For citation, three approaches are possible:
Cite a time slice (the set of updates to the dataset made during a particular period of time);
Cite a snap shot (a copy of the entire dataset made at a specific time);
Cite the continuously updated dataset, but add an Access Date and Time to the citation.
Examples: DOI assignments on datasets: doi:10.1594/WDCC/MODIS__Arctic__MPF , doi:10.5676/DWD_GPCC/CLIM_M_V2011_100
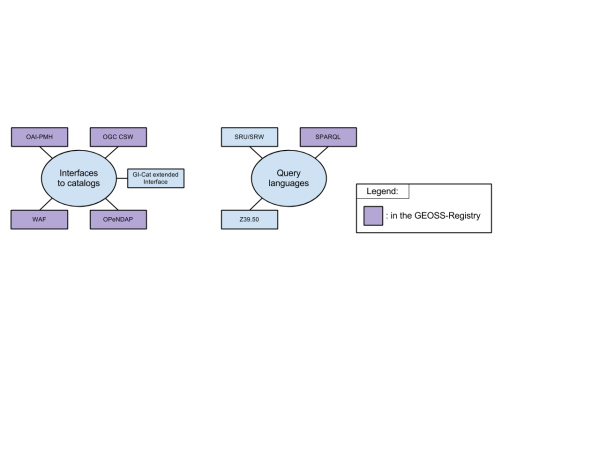
Data Ingest examples
Data download examples
CERA WWW-Gateway - Jblob Version 2 http://cera-www.dkrz.de/CERA/jblob/
CERA GUI http://cera-www.dkrz.de/WDCC/ui/
ESGF
Access: http server, GridFTP and OPENDAP
Interface more resources, submissions systems